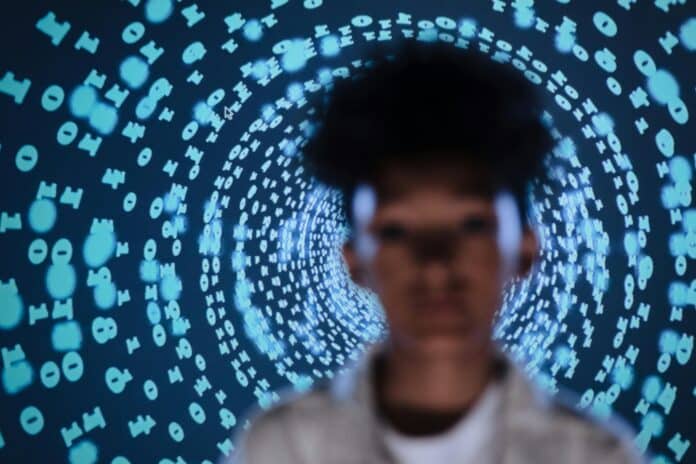
1. The Shaky Heart of the Machine: Qubit Stability
If classical computing is built on bricks, quantum computing is built on smoke. Qubits—quantum bits—don’t stay put. They drift. They decay. They throw tantrums at the slightest noise, like toddlers with doctoral degrees in physics.
Stability isn’t a feature here. It’s a fantasy developers chase with absurd precision. One stray photon, one vibration, one whisper from the air-conditioning unit—and coherence collapses. Decoherence, they call it. A polite word for system failure.
In the Quantum AI world, this fragility is a killer. You can’t run machine learning algorithms on a system that forgets what it was doing halfway through a calculation. So, we build error-correcting codes. We stack redundant qubits like sandbags against the flood. We freeze hardware to near-zero kelvin and pray the silence holds.
And yet, even with the best tech—ion traps, superconducting loops, photonic grids—we’re still measuring success in microseconds. It’s not elegant. It’s barely usable. But this is the bedrock. Get qubit stability wrong, and everything else is a theory scribbled on a blackboard.
2. Scaling Up: When More Isn’t Enough
Everyone wants more qubits. The headlines talk about 50, 100, 1,000. But it’s not about quantity—it’s about control. Most quantum processors can’t keep their qubits stable long enough to do anything meaningful. Scaling that chaos? That’s not engineering. That’s warfare.
Quantum AI relies on these systems being just reliable enough to run models—classifiers, optimisers, neural nets—without collapsing like a flan in a cupboard. But the larger the system, the louder the noise. Interference scales like debt in a bad economy.
The challenge isn’t just adding qubits. It’s linking them without inviting more error. Building logic gates that don’t spill. Running algorithms that won’t buckle under the weight of complexity. Companies like IBM, IonQ, and Rigetti all make noises about roadmap milestones—but behind the gloss, they’re fighting thermal noise, material defects, and physics itself.
The dream is a fault-tolerant, universal quantum computer. The reality is a stack of prototype boards, half of which crash before boot. And yet, somehow, progress inches forward. Not with fanfare—but with duct tape, data, and repetition.
3. The Role of Quantum AI Amid the Wreckage
Let’s be honest: Quantum AI isn’t arriving on a chariot of fire. It’s limping through the lab, leaning on approximations. Most systems running “quantum machine learning” today are hybrids—classic hardware doing the bulk of the work, with a quantum co-processor chipping in where it can.
Still, there’s promise. Quantum AI algorithms—quantum kernel methods, variational quantum circuits, quantum Boltzmann machines—are being developed with one eye on the future and the other squinting through hardware limitations. They don’t need thousands of qubits to provide value. Sometimes, a handful, wielded wisely, is enough to detect non-linear patterns classical systems miss.
But don’t confuse research momentum with real-world impact. The majority of quantum AI research happens on simulators. Theoretical environments that pretend qubits behave, while real ones collapse like underfunded startups.
This is not the stuff of moonshots. It’s the grim slog of foundational science. And still, it moves.
4. Quantum AI Trading: Speculating on the Edge of Chaos
Markets love volatility. Quantum AI doesn’t just tolerate it—it speaks the same language. The financial sector, for once, is ahead of the curve—not out of curiosity, but out of desperation for an edge.
In trading, uncertainty isn’t the enemy. It’s the game. Quantum algorithms exploit this, handling tangled variables—pricing derivatives, modelling risk, detecting market signals buried in noise. Quantum-enhanced Monte Carlo methods, quantum annealing for portfolio optimisation, and entanglement-based correlation analysis are slowly creeping into quant labs.
Startups like Multiverse Computing aren’t trying to reinvent finance. They’re trying to carve out milliseconds—those slivers where fortunes shift. Their pitch: get insights faster than the next guy, or get out of the market.
But let’s be clear—this isn’t open access. It’s not a democratising force. Quantum AI in finance is a scalpel for the elite. And the first to wield it effectively will not be writing Medium posts about it. They’ll be watching your trades, two steps ahead.
5. The Long March: What Progress Really Looks Like
The narrative’s tempting—supercomputers cracking world hunger and disease by 2030. But Quantum AI isn’t cooperating with that timeline. It moves like geology. Quiet. Relentless. Often invisible.
Progress looks like better noise filters. Longer coherence times. Slightly fewer errors per operation. These aren’t viral breakthroughs—they’re technical footnotes. But each footnote stacks. And eventually, the stack might matter.
In the meantime, the field lives in limbo—too important to ignore, too immature to monetise. Researchers publish cautiously. Investors sniff around. Startups over-promise and quietly course-correct.
But there’s no turning back. The classical paradigm is tired. The problems we’re dealing with—climate modelling, drug discovery, cryptographic collapse—aren’t waiting for permission. If we’re lucky, quantum AI catches up before it’s too late.
FAQ: No Illusions, Just Answers
Why do qubits need to be so cold?
Because thermal noise ruins everything. To keep qubits in a stable quantum state, they must be cooled to near absolute zero—where atoms barely move. Think expensive freezers and power bills that hurt.
What’s so hard about scaling quantum computers?
Each new qubit adds more potential error. You don’t just add processing power—you add complexity. The wiring, calibration, and environmental control needed to keep even 100 qubits stable is absurd.
Is Quantum AI actually useful today?
In small, specific cases—yes. Most use-cases are still experimental or rely on hybrid models. We’re not solving global crises with it yet, but the research is laying groundwork.
Why does finance care about Quantum AI?
Because markets are noisy and unpredictable. Quantum AI handles complex probability distributions better than classical systems in theory—so hedge funds smell blood.
Where can I find more without the hype?
Try Quantum AI. It cuts through the fantasy and tells you what’s real. Or at least what’s becoming real.
Photo by Ron Lach : Pexels